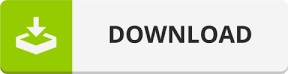

- #Connect to mongodb how to#
- #Connect to mongodb update#
- #Connect to mongodb code#
- #Connect to mongodb download#
To verify our Spark master and works are online navigate to Figure 4: Spark master web portal on port 8080 Creating a moving average using PySpark
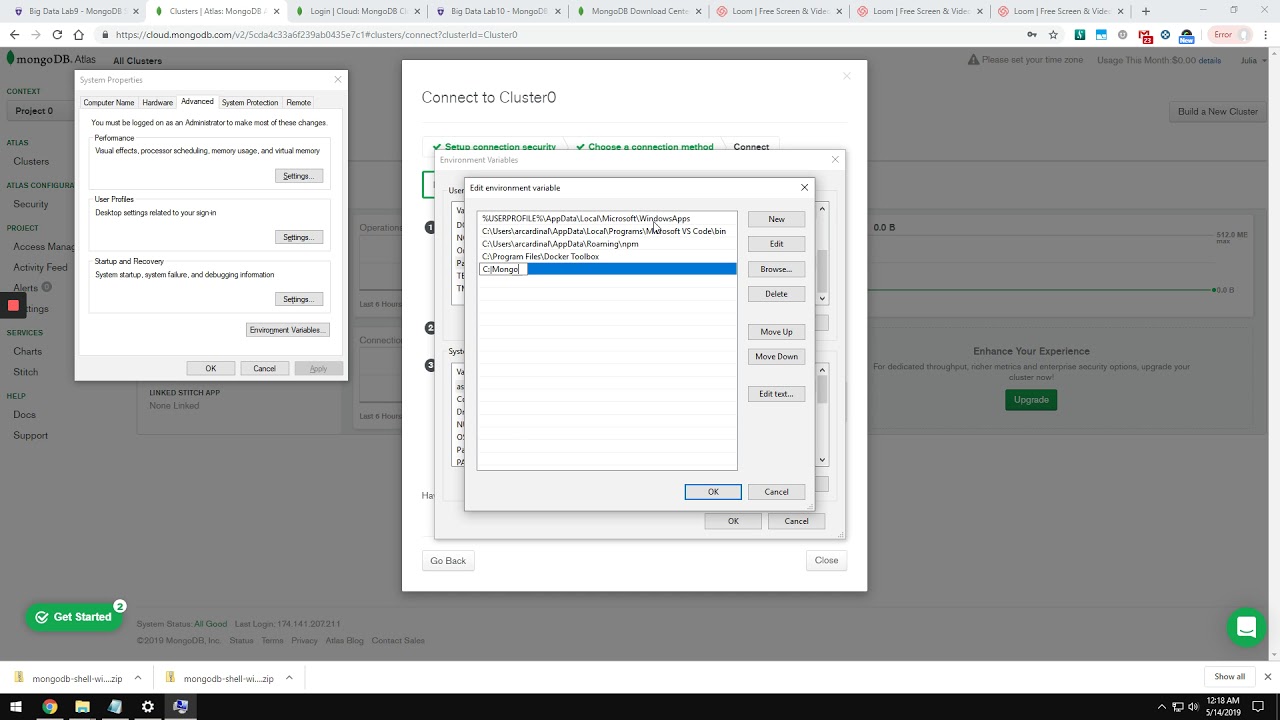
To verify our MongoDB cluster is up and running we can connect to the default port 27017 using the mongo shell.įigure 2: Mongosh shell tool connecting to the MongoDB clusterįinally, we can verify that the Jupyter Lab is up and running by navigating to the URL: Figure 3: Jupyter Lab web portal
#Connect to mongodb download#
To download this new visit the online mongo shell documentation. Mongosh addresses some of the limitations of the original shell such as syntax highlighting, auto-complete, command history, and improved logging to name a few. At the time of this writing, there is a new version of the MongoDB Shell called mongosh that is currently in Preview. The mongo shell command line tool has been the de facto standard since the inception of MongoDB itself. To interact with MongoDB there are a variety of tools options. The MongoDB cluster will be used for both reading data into Spark and writing data from Spark back into MongoDB. Spark is also deployed in this environment with a master node located at port 8080 and two worker nodes listening on ports 80 respectively. The run.sh script file runs the docker compose file which creates a three node MongoDB cluster, configures it as a replica set on port 27017. To follow along, git clone the RWaltersMA/mongo-spark-jupyter repository and run “sh build.sh” to build the docker images then run “sh run.sh” to build the environment seen in Figure 1. Let’s start by building out an environment that consists of a MongoDB cluster, an Apache Spark deployment with one master and two worker nodes, and JupyterLab. The docker compose scripts used in this article are based on those that Andre provided in his article. A special thanks to Andre Perez for providing a well written article called, “ Apache Spark Cluster on Docker”.
#Connect to mongodb code#
While you can read through this article and get the basic idea, if you’d like to get hands-on, all the docker scripts and code are available on the GitHub repository, RWaltersMA/mongo-spark-jupyter.
#Connect to mongodb update#
We will load financial security data from MongoDB, calculate a moving average then update the data in MongoDB with these new data.
#Connect to mongodb how to#
In this article, we will showcase how to leverage MongoDB data in your JupyterLab notebooks via the MongoDB Spark Connector and PySpark. Spark works efficiently and can consume data from a variety of data sources like HDFS file systems, relational databases and even from MongoDB via the MongoDB Spark Connector. This is the technical implementation of the english saying, “many hands make small work”. The key concept with Spark is distributed computing taking tasks that would normally consume massive amounts of compute resources on a single server and spread the workload out to many worker nodes. Spark is an open source general-purpose cluster-computing framework that is one of the most popular analytics engines for large-scale data processing. This new web-based interactive development environment takes Jupyter notebooks to a whole new level by modularizing the environment making it easy for developers to extend the platform and adds new capabilities like a console, command-line terminal, and a text editor.Īpache Spark is frequently used together with Jupyter notebooks. The Jupyter notebook has now evolved into JupyterLab. A simple web UI that makes it simple to create and share documents that contain live code, equations, visualizations and narrative text.
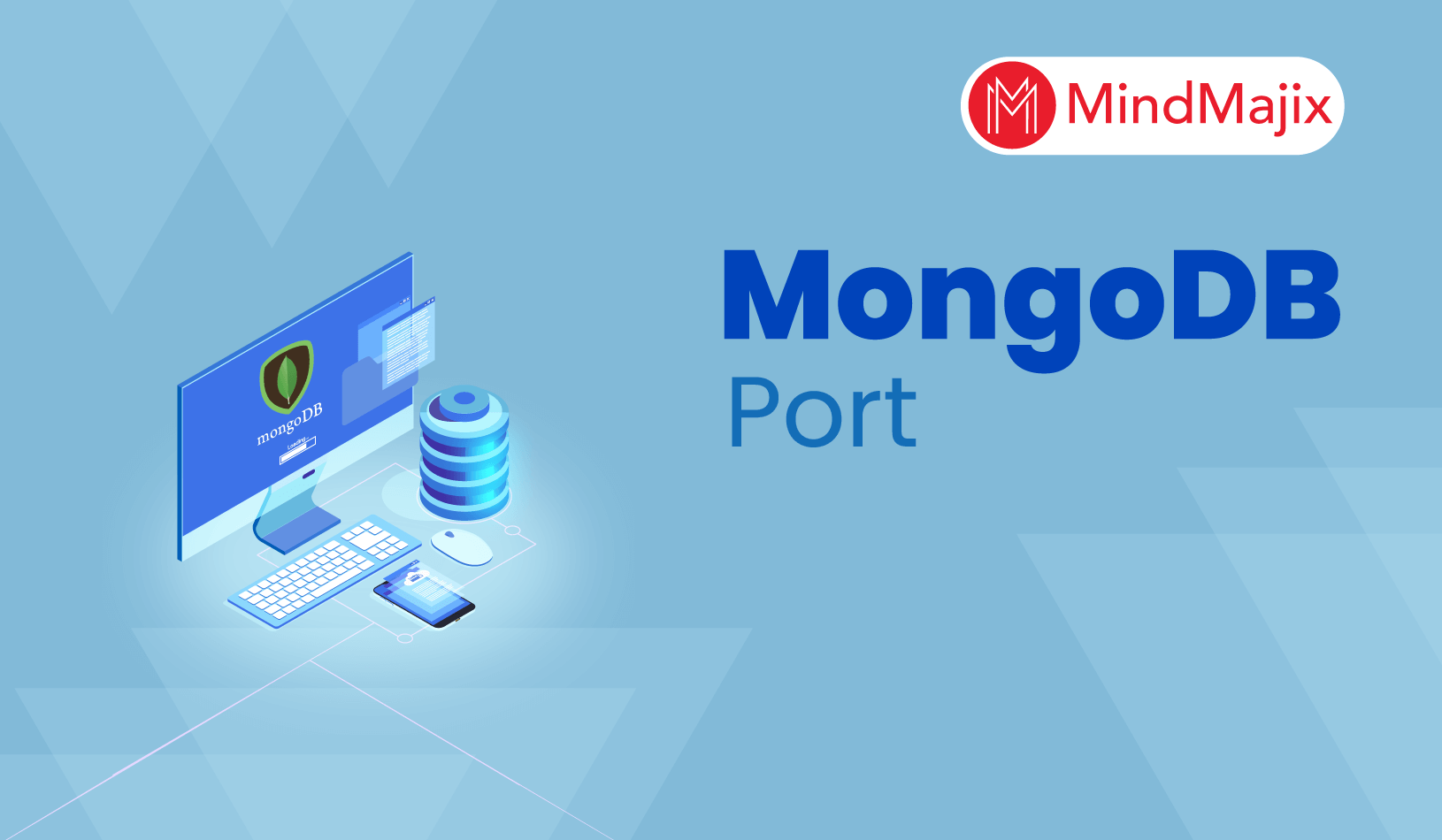
Jupyter notebook is an open source web application that is a game changer for data scientists and engineers.
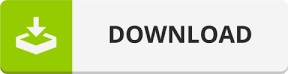